
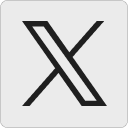

This week’s podcast is about Huawei’s AI strategy. Specifically it’s “Cloud for AI” approach to hardware and infrastructure.
You can listen to this podcast here, which has the slides and graphics mentioned. Also available at iTunes and Google Podcasts.
Here is the link to the TechMoat Consulting.
Here is the link to our Tech Tours.
Here is the Huawei Cloud Computing document I mentioned. Here.
Here is the overall AI Cloud strategy (from Huawei Twitter account):
Here are the mentioned big trends. Sorry I can’t post the slide.
- Pre-training scaling
- Post-training scaling
- Inference-time scaling
- Distillation scaling
- Multi-agent scaling
Here are the 6 pillars (i.e., differentiating factors).
- Be the customers’ choice for security, stability and quality.
- Create a global infrastructure with high availability and low latency.
- More customers to choose Huawei Cloud’s cloud-native, AI-ready infrastructure.
- Enabling a vast range of AI models to reshape industries.
- Build knowledge-centric data foundations with data-AI convergence.
- Synergistic innovation across cloud, network and device.
——-
Related articles:
- Lessons from My Visit to Alibaba Cloud (Tech Strategy – Podcast 244)
- AutoGPT: The Rise of Digital Agents and Non-Human Platforms & Business Models (Tech Strategy – Podcast 163)
- Why ChatGPT and Generative AI Are a Mortal Threat to Disney, Netflix and Most Hollywood Studios (Tech Strategy – Podcast 150)
From the Concept Library, concepts for this article are:
- AI Cloud
From the Company Library, companies for this article are:
- Huawei
——transcript below
00:05
Welcome, welcome everybody. My name is Jeff Towson and this is the Tech Strategy Podcast from Techmoat Consulting. And the topic for today, the six pillars of Huawei’s cloud for AI strategy. Now I was recently at a Huawei event in Phuket, which is pretty cool. I’ll talk a bit about that. Looking at what they’re doing in AI cloud and
00:32
This is really, think, the most exciting thing they’re doing. This sort of, how would you build cloud architecture, everything from databases to servers to chips to all of that, that is sort of built for AI first. And they call that cloud for AI and it goes hand in hand with their other strategy, which they call AI for cloud, which is where they’re doing models and other sorts of things. But, you know, I think the infrastructure piece is where they’re strongest.
01:28
But apart from that, the business side and the tech side is really pretty cool. So, I’m going to kind of summarize what I learned about that over the trip. Anyways, that will be the topic for today. Housekeeping stuff, we have two China tours coming up basically in October. One is going to be the urban tech tour, which is going to be looking at urbanization, smart cities, green cities. How do you apply technology?
01:57
to city design, city structure, which is relevant for investors, real estate people, sovereign wealth people, and that sort of thing. And arguably, China’s the best at this in the world. They build more cities than anybody, faster, in many cases better, not 100%, but a lot of cases. So that’ll be in October. The other one we’re going to do is sort of a China tech tour, e-commerce focus, retail focus. That’s really for retailers, merchants, brands, CPG.
02:24
anyone who’s trying to sell anything online for the most part. And again, I think China’s arguably the leader when it comes to e-commerce and retail going digital. If they’re not the leader, they’re pretty close to it in most sectors. So anyways, if you’re interested in that, go over to Tecmo Consulting, you’ll find the details for the two tours are there, or just send me a note, can talk about it.
02:47
Okay, standard disclaimer, nothing in this podcast, my writing website is investment advice, the numbers and information from me and any guests may be incorrect. The views and opinions expressed may no longer be relevant or accurate. Overall, investing is risky, this is not investment, legal or tax advice, do your own research. I’m getting faster at that, I think. Okay, let’s get into the topic. So, the topic for today, the concept for today, there really isn’t one, it’s just AI meets cloud, which.
03:15
For me is ground zero for everything. It’s the area I’m most focused on is, know, because AI is obviously the biggest type of transformation disruption happening. Most companies are building that off the cloud to begin with. And then they’re building things more natively. They’re adopting models for their own businesses. But, you know, the cloud is kind of a reasonable first step for virtually everybody. So, AI focused cloud is where I’m kind of focusing. What are the major companies building?
03:44
which in Asia, know, Alibaba, Tencent, Baidu, and I think Huawei’s on that list. That’s China for sure, but also APAC. You know, this is where we see a lot of action. You also see Google here, you see AWS, but I think it’s the sort of China-based companies that are in many ways the most aggressive and who are sort of viewing this market the most strategically. They have to win Asia.
04:11
It’s optional if you’re a US cloud company like Google. It’s a must-win for a China-based company. So, lots of action happening in this space. Okay, so this conference, which was down in Phuket, which is pretty fun island, kind of crazy these days. The conference was based on Huawei Cloud for APAC. So, sort of non-China. So, a lot of people from Hong Kong, well, I mean, that’s kind of on
04:40
both there, but a lot of people from Singapore, Malaysia, Philippines, Indonesia, Thailand, everyone going there to learn sort of what’s going on. And it was more focused for partners, so resellers, consultants, software integrators, basically the people who sell these services to a large degree. interesting, and it was mostly about AI, and it goes into two buckets, cloud for AI, AI for cloud.
05:07
Cloud for AI is sort of hardware focused. AI for cloud is more software where AI is the focus. In Huawei, to be fair, it’s not 50-50. They’re 75-80 % on the first. I they’re really good at hardware. They’re really good at everything from edge devices, smartphones, smart cars, smart homes, to connectivity, 5G, now 5GA, and then cloud, which…
05:34
AI usually sits mostly there, but it can often even go down to the edge computing level too. So, they can address the whole hardware ecosystem, which most companies cannot do. Google can’t do that; Amazon can’t do that. They’re much more of just cloud and AI. But Huawei’s going for both, the software and the hardware, and they’re quite strong on the hardware side. So, cloud for AI as opposed to AI for cloud. I think that’s…
06:02
That’s what they’ve got going right now that’s the most compelling. And that’s what I’m going to talk about. As for Phuket, pretty fun place. I had a good time. I’ll talk about it at the end. In terms of the conference, there were really two presentations that stood out to me. The first one was a guy named Joy Huang. I’m going to talk about basically his talk today. And he’s the strategy guy for AI and cloud and really very, very smart.
06:30
He thinks a lot like me actually that’s kind of probably why I enjoyed it so much. He comes at strategy very much the way I do though I thought that was quite cool. And then there was another guy who was their chief product officer whose sort of building everything that they’re doing so that was very helpful. I’ll talk about that in a separate podcast or an article. I’m going to write this up as well so I’ll send it out to subscribers. But this talk is mostly about Mr. Huang, Joy Huang who’s you know the
06:58
industry and strategy guy. Very, very interesting. And this is the strategy group that wrote a pretty big, I would say book. It’s more of a book than a document. And it’s all published and it’s basically called Cloud 2030, Cloud Computing 2030. It’s this great document about how they’re thinking about the subject and what they’re doing. I’ll put the link in the show notes if you want to download it. It’s free. It’s on the Huawei website.
07:28
They call it a white paper, kind of looks like a book. Anyways, really pretty good reading and that’s his team that did this. And so, a lot of what they’re doing cloud-wise, strategy-wise comes from them. so first point which I think he made that was compelling was, okay, AI performance versus computing power.
07:58
And there’s this idea of if we throw more computing power, does the AI get smarter? And for a lot of the last two years, that’s been the go-to solution. And you hear this in the news all the time. Grok is building the colossus massive server farm with 100,000 or 200,000 GPUs. Therefore, its AI is better. It has better performance.
08:26
And that was kind of what we’ve been hearing for a long time, to say if you ramp up the number of chips, it’s going to get better. Now DeepSeek kind of came at that differently and said, hey, you can get most of the power with a much simpler architecture with a lot less compute, which by the way is a lot less expensive. Okay, he came out with an argument that basically said, look, there’s five scaling laws here that happen one after the next. As you increase the compute power, you get better performance,
08:56
in a certain area and that would be a scaling law and you can kind of go from scaling law one to two to three to four to five over time but generally we’re going to see non-linear increases in performance with more and more compute power based on these five laws. So, law number one paradigm number one he calls it just pre-training scaling. This is kind of in the last couple years the more GPUs and servers we throw
09:25
the faster and better the training becomes, which is largely true. And when you look at compute power, training requires far less. Ongoing inference requires a lot more and it’s a lot less predictable. But you can kind of put boundaries on, we’re going to train this model and as we’re getting better at this, the training is happening faster with better performance, with better accuracy and so on. So, pre-training scaling.
09:54
Okay, we’ll call that 2023, 2024. And paradigm two and three would be basically post-training. So, paradigm two is post-training, paradigm three is inference training or inference scaling. And yeah, I would put all that into the last year or so and probably this year as well. Okay, none of that’s terribly interesting. What was interesting was four and five. Number four is distillation scaling.
10:23
That’s a really interesting subject. If you haven’t looked into sort of distillation.
10:29
That’s really, really cool and that’s a lot of what DeepSeek is doing. And it’s, I’m going to paraphrase this badly, but it’s this idea of you can take the expertise of a model and distill it down into a usable playbook that a much smaller model can use. So, it’s like, if we want to train a chef, that’s a full skillset. We are creating a professional world-class chef.
10:58
fine, that would be a foundational model. However, at the same time, that chef can kind of write a recipe book and we can distill the expertise of the chef into a recipe book. And we can give that recipe book to a simpler, smaller model. That’s distillation. And that’s a lot of what DeepSeek was doing so that when they launched and OpenAI complained,
11:23
They kind of said, look, you just copied our model and distilled it down into something simpler that has 80 % of the performance, much, much cheaper, which was a decent argument. And the counter argument to that is, well, yeah, but where did you get all the data to train your massive model? Didn’t you kind of steal that from everybody’s web pages? Didn’t you kind of scrape the entire web and all of YouTube and all of that? So, let’s not, know, who stole from who? I don’t think.
11:52
We don’t call this stealing; this is something else. Anyway, so distillation scaling, it’s kind of where we are now. So, paradigm four is interesting, then paradigm five is multi-agent scaling. Okay, we create an agent. What’s the difference between an agent and AI? The way I view it, again, I’m paraphrasing simply, I view it as an AI that can make decisions on its own and can take actions because it has a toolkit.
12:21
You want to turn an AI into an agent, give it the authority to not just predict what should happen, but then to make the decision. And give it a toolkit that it can draw on to take action. So instead of recommending a pizza for me to order tonight, I want the agent to actually do it. Well, it makes the prediction, this is a good pizza for Jeff. Then it can take action. It can make the decision; we’re going to buy a pepperoni pizza or whatever.
12:50
then it can take the action by accessing its toolkit, is, it can go in and do payment, it can go into the Domino’s website, but these AI agents are getting a big suite of tools, and this is why the MCP protocol is so helpful, because it gives an agent really an almost unlimited toolkit it can reach into. So, the agent starts to look like us going from website to website, using whatever tool we want to order our pizza, send an email, buy a book.
13:19
whatever, the same way we can go web browser site to site, it can go from tool to tool to get whatever it wants to do, what it wants to do. That’s an agent. Okay, paradigm five, multi-agent scaling. This is when you’re not deploying one agent to do what you want. Maybe you deploy 10. Maybe you have an agent that’s really good at doing legal stuff. Maybe you have an agent that’s really good at shopping online.
13:46
Maybe you have an agent who’s really good at doing accounting and doing the back office. Maybe you have an agent that’s a sales agent for your business. Let’s deploy 10 agents to do what we want to do. And both of these distillation scaling and multi-agent scaling should get you an exponential return in performance based on more compute. So, we add a little bit more compute, but if we’re tapping into those,
14:15
It’s not going to go up linearly, it’s going to go up exponentially in terms of AI performance. I took a photo of his slide, I’ll put it in the show notes, but it basically shows these five paradigms. Pretty impressive, pretty compelling. I don’t think that’s the whole story, I think it’s one opinion. But yeah, I tend to be on board with the current focus on inference, distillation’s a big deal, and then, you know, let’s not even talk about agent-based scaling.
14:44
Let’s go right to multi-agent, right? Let’s just dump to the fact that, and then you start to get into things like AI swarms, which they talk about with robotics. Let’s deploy 100 agents. Let’s deploy 1,000 agents, right? You can see that that’s, we’re not going to stick with using one agent for very long. We’re going to have armies, teams, management teams, and so on. Anyway, so I thought that was pretty interesting, and I like people when they make these sort of pretty, if you’re going to do strategy,
15:13
You know, I’ve heard strategy described as big wave riding. I think it was one of the all-in guys said that, or investing if not strategy. And I like that idea a lot. So much of this is about finding the right wave to ride and not a little wave, the big one. And once you’ve made that decision, all the other strategy decisions, how are we going to beat our competitors? How do we deal with new entrants? All that stuff. How do we make our customers happy? That’s all secondary.
15:42
If you can identify a really big wave to ride, everything becomes easier. So that’s kind of how I viewed that part of the presentation. Look, he’s laying out the waves he sees coming in the future. okay, compelling. I’m pretty much on board with that. right, so with that, let’s get to sort of the Huawei strategy. If those are the waves to ride, let’s talk about the strategy. And that’s basically, you know.
16:09
kind of what I said, cloud for AI and AI for cloud. So, Huawei, their cloud business is basically said we’re doing hardware and we’re doing software.
16:20
Now they have all these announcements. There are the chips, there are new servers, there are data lakes, there are data oceans, which I’ll talk about. And then all the models you hear about all the time. Pangu, they’re integrating DeepSeek. mean, they have a whole ton of stuff going on. But you can put it into one of those two buckets. Look, it’s cloud for AI. The idea that we’re going to do lots of innovation at the system level, at the architecture level, at the hardware level. And they’re very, very good at this.
16:49
And then there’s AI for cloud, which is more about, okay, what are the services you can use in your business? How do we reshape and innovate in terms of services we offer? And all of that sits on the hardware. Okay, they’re kind of doing it in both ways. And against those two buckets, they basically have six pillars. Three pillars go under cloud for AI, three pillars go under AI for cloud. So, the first three are, they’re about hardware, mostly.
17:18
The second three are more about services and data and things like that. So, I’m going to go through them. The way Mr. Huang talked about it, he basically said these are the differentiators. If you’re doing strategy, question number one, what wave are we going to ride? Question number two, how are we going to be different than everybody else? So, you lean into your strengths, you learn into what you can do, creates a better value proposition, maybe it’s stuff others can’t do as well as you, or they can’t do it all.
17:46
So, he basically outlined six areas to differentiate with an AI cloud and this is what I’m calling their six pillars And I’ll read them off to you and then I’ll go through them. But basically, number one better security stability and quality in the hardware side so cloud for AI And security is obviously a big deal, especially if you’re selling cloud services and servers and data lakes if you’re selling that to a bank Security is a huge deal
18:17
Right? So that’s number one. Number two is global infrastructure that has better latency and availability. This is actually in my book, one of the two really cool things they’re doing. And I’ll talk about that. They’re building basically networks of cloud servers all over China, increasingly all over Asia. And it’s going to go by that you can access to run your AI with lower latency, better availability, because like they’re better at this stuff. They’re great at hardware.
18:47
That’s number two, I think that’s a big one. Number three is the other big one in my opinion, which is they’re calling it cloud native services. I call it kind of the cloud matrix, which is really this idea that we’re going to have architecture that is fundamentally built for AI, which is different than saying we have a lot of servers, we have cloud-based servers, we have on-premises services that are based on CPUs for the most part.
19:15
And we’ve been adding GPUs and trying to adapt this to some degree to make it work with AI. No, we’re going to build architecture that is from the chip level up, native for AI.
19:45
So that one’s pretty important. this number two and number three are the most important. Number four is sort of foundational AI models and tech. So, this is lots and lots of models. Now we’re in AI for cloud. They’ve got a whole huge suite of models they’ve been building and it works with external models as well. Foundation models, LLMs, all that. Number five, data plus AI convergence. This idea that…
20:12
It’s not like you have data and then the AI runs off the data. Because you kind of have to shape the data. You can’t just dump it into a database anymore like it’s easily quantifiable like a spreadsheet. No, the data’s getting messy. You need to combine AI with data at the data layer so that it is more usable. And they’re calling that basically, look, we’re going to convert
20:41
our data layer, our data lakes into intelligence themselves. And then we’re going to draw on that with our models. But we’re going to start integrating in AI and data, they call it the convergence, at the data layer to create sort of a knowledge-centric database as opposed to just these massive data lakes and these data warehouses. So that’s kind of interesting as an idea. Not sure how much I buy it, but kind of interesting. And then number six, this idea of
21:11
architecture innovation where they can connect everything cloud to network to device in one system, which really very few others can do. And if you’re running AI cloud for let’s say a railroad or a port or a mining facility, having all of those things integrated tightly can be a big deal. You’re running the…
21:36
the cranes on the port in real time from your AI because it’s all tightly integrated. And some of the AI is running in the cloud, some of it’s running on the crane, and you’ve got 5G, really 5GA connecting this thing at tremendous speed. It all becomes one thing. So that’s number six. So, number one, which I’ll just, I’m going to put the slides in the show notes. There’s basically one slide for each of these.
22:02
pretty good slides actually. Huawei does, I don’t know who does this at Huawei, but somebody there loves to jam as much text on a slide as possible. So, they’re almost like reading books and I really like it because I can take photos of them and I go through them later. But if you’re actually trying to watch the presentation, it’s just all these words go by. somebody’s deep into like, let’s jam text into PowerPoint slides at that company.
22:30
Anyway, so number one, I’ll go through them in more detail. Number one, as a differentiator, as a key pillar of the AI strategy, this goes under cloud for AI hardware focused innovation. The customers become basically the customer’s choice for security, stability, and quality. Okay, that’s a pretty good pitch to customers. And it’s not just cloud, but really what you’re talking about is the mainframes in the company.
22:57
that also connect with the cloud, because most everyone’s running hybrid anyways. Okay, how do you keep that secure? Big deal if you’re a bank. How do you make it stable? How do you know that there’s going to be zero accidents, zero downtime? Things like that, and then also quality. What kind of products are you running? How good is the engineering? How good is your hardware? All of that stuff. So, if you want to get into the hardware side of this,
23:26
You can look up their quality management system, is called E2E. A lot of cybersecurity stuff, a of privacy protection stuff, fault alerts, data security, account governance, verification changes. mean, there’s a whole world of published literature on this whole subject. It’s a big deal. That’s worth doing. So, number one, that makes a lot of sense. Number two, which I think is kind of the exciting one.
23:56
Global infrastructure with high availability and low latency. Okay, that’s cool. That means they are going for a cloud network. So basically, putting data centers and servers all over the world that anyone can access global infrastructure that is highly available and low latency, which means you can access it really, really quickly with low latency, which…
24:24
basically, means the servers have to be very close by. And the term they use for this, which I think is a better description, is they’re basically building a distributed cloud infrastructure. So, 33 regions in the world that are going to be, have basically this sort of infrastructure available. They’re calling it availability zones, regions, but basically covering the whole world with a distributed cloud computing.
24:54
Structure that anyone can access and the terms they use which I think are actually pretty cool They talk about a cloud ocean versus a cloud sea versus a cloud lake Now people know what a cloud lake is. Okay. Here’s your data lake. That’s what you’re accessing. That’s close to your company probably the access couple milliseconds five ten in that range That’s for stuff. That’s high demand. So, cars
25:24
autonomous vehicles, manufacturing facilities, they’re all going to have to access the cloud lake fairly quickly. It’s going to have to be close by. The other extreme would be the cloud ocean. This is where you have, let’s say in China, three major facilities, could be four, could be five, looks like it’s in that range, that cover the whole country. They’re going to be in regions that are cold.
25:53
big, massive data server farms in Guian and other places that are cold and have a lot of wind easier to keep cool. So, you’re going to have these cloud oceans, massive servers, massive ability to compute, but the latency is going to be 50 to say 100 milliseconds.
26:13
So, it’s going to be sort of this distributed architecture. In between the cloud ocean and the cloud lake, you’re going to have the cloud sea. So, this is a couple hundred, let’s say 100,000 servers, 150,000 servers, smaller sites, going to be a lot closer to the major cities.
26:32
speed latency is going to be 10, 15 milliseconds. So, you’re starting to see this sort of distributed cloud architecture that they’re building in China, now in Asia, but as far as I can tell, they want to go everywhere. And they’ve mentioned, you know, they’ve got some facilities out in the Middle East, across Asia that are going. So that’s really interesting.
26:57
this idea of a global cloud architecture. Now as a strategy guy, I like that, because that looks to me like something that’s very, very difficult to replicate. Now you could try and replicate that, but can you match their latency times? Can you match their stability? Can you match their security? Can you match the availability? Well, this is a game of economies of scale. This looks to me like a massive, tangible asset that the leaders with economies of scale
27:26
are going to have superior performance versus everybody else. Okay, that looks like good strategy to me. Okay, and that brings us to what I think is kind of the most exciting thing. Number three, basically a sort of cloud native AI ready infrastructure. You’ve got all these servers everywhere. You want to offer infrastructure as a service and then you want to offer models and you want to offer your AI services. Okay, fine.
27:56
What are the chips that are in all of these massive data farms that are being built globally?
28:51
That’s the big question. What is in all these servers and all these data centers that are going to create this global distributed cloud structure? Okay, then we get to the cloud matrix. What is the cloud matrix? Okay. You know, it’s been CPUs, lots and lots of CPUs, and then people have started to add GPUs. And the GPU is obviously very, very powerful. That’s most of what’s getting you the sort of usage of the AI.
29:20
Okay, the question is, and this is me paraphrasing my understanding, which is probably 50 % at best. Can we sort of pool together GPUs, CPUs, DPUs, NPUs, and memory chips into sort of a matrix?
It’s a composable, connected mix of CPUs, GPUs, DPUs, MBUs and memory. Can we get that to work, which we call the cloud matrix? Which is the semiconductor area.
33:10
We’ll see, but it sounds like we’re kind of right there. Anyways, that’s number three, this idea of this cloud native AI ready infrastructure that they’re building out, which goes sort of hand in hand with this distributed computing architecture that they’re building globally. Pretty cool. Okay, those are the two main points for the day. The others I’ll go through more quickly, but those are the ones I’m thinking about a lot. Okay, so number four.
33:39
One, two, and three are about cloud for AI. Four, five, and six are about AI for cloud. This is more software services, AI services that people can use. Okay, number four, this is their language, enabling a vast range of AI models and reshaping industries. Okay, the English is a little spotty, but the key word here is a vast range of AI models. They’re like Baidu, like Alibaba Cloud. They are playing across the board.
34:08
They are building AI models, foundation models for everything. Now, I think the two things that make them a bit different, and number one, they’re industry focused. That’s actually very similar to Baidu and Alibaba Cloud. They’re building models that will get better and better for specific industries, banking, transportation, manufacturing, I think are the biggest three. They’re industry focused.
34:36
You don’t get the sense that OpenAI is super industry focused. They are. Number two, they’re building these in basically three levels. So, you have the L0, the foundation models, their multimodal models, their research models, their video generation models. Fine, these are general purpose L0 level models. Then on top of that, you have the L1 industry specific models.
35:04
And in theory, you get the intelligence feedback loop. The more people that adopt the manufacturing model, the smarter the intelligence of L1 will get. Then you get up to L2. This is the more scenario-specific models, chatbots, customer service, billing, things like that. So, they’re doing L1, sorry, L0, L1, L2. That’s a little different than what we see at companies otherwise. Very interesting. I won’t go through all their models. I think you’ve; I’ve talked about these before.
35:34
The Pongu 5.0 model is out. That’s their in-house models or the Pongu models. So, they have 5.0 which is now out. They have their edge version. These are small models, medium long models, super big models. They can be run in the cloud. They can be run hybrid and so on and so on and so on. I won’t go through all their models. It’s a big, long list.
36:03
But also, I guess the key thing here is their architecture, their infrastructure, everything I just said about distributed cloud, you don’t have to use their models. You can use DeepSeek, you can use Llama, you can use Quinn, you can use all of them. So, Huawei has always been much more in the infrastructure business than any particular product. I do think it’s interesting how much DeepSeek is mentioned as part of the Asia.
36:32
AI picture that Chinese companies, Chinese software developers, resellers, software consultants are really talking about DeepSeek in Asia, Thailand, Malaysia, Singapore a lot. DeepSeek is really kind of an amazing phenomenon of how much adoption it’s getting all over the world so quickly.
36:55
That’s number four. Number five, fifth pillar. This is the one I mentioned before, which is this idea we’re going to converge data and AI. And we’re going to shift from things like data lakes to knowledge lakes. So, they titled number four, building knowledge-centric data foundations with data AI convergence. That all of these models, we shouldn’t be building them on just data lakes sitting in Excel spreadsheets.
37:24
which we’re now combining, well that’d be a warehouse, now we’re combining with all sorts of videos and unstructured data, no, no. We should combine data lakes with AI and start building knowledge lakes that are specific to industries. And that’s what the model should be running on. Okay, the argument they make, which I don’t know if they’re right, they basically say, look, when you combine data and AI, you get knowledge lakes and that gets you.
37:52
far greater utilization of resources. It gets you 10x data supply efficiency. It’s just a more usable form, so you’re going to get much better performance, you’re going to get much better efficiency. Okay, maybe, I’m open to it, I don’t know. It’s beyond me in terms of technical knowledge. But we’ll see. Anyways, then number six, last one.
38:18
Synergistic innovation across cloud network and device. Okay, this is the standard Huawei end-to-end solution. We can offer you cloud; we can offer you connectivity, and we can offer you edge computing. We make smartphones, we can put these things into cars, we can put these things into mining devices, we can do the 5G private network that you can connect with all your mining devices.
38:44
and we run the cloud and the AI there. But the AI is going to run across all of these synergistically. So, their language here is pretty interesting. Synergistic innovation across cloud network and device. That’s pretty interesting. We’ll see. But obviously, they’re going to lean into that. And they’re going to lean into that in industries where an end-to-end solution is going to be more powerful.
39:13
Now none of that helps you if you’re just using AI, I don’t know, to redo your billing for your office. Let’s put an AI agent in who checks all the billing and the claims. Okay, at least for the billing part, we don’t need all of that. But if we’re going to take our port, let’s say in Thailand, or a smart mine in China.
39:37
and we’re going to integrate the whole thing and have remote mining devices deep underground that are either operating autonomously or they’re being controlled remotely by miners on the surface sitting in cabs controlling them. Okay, we need all of that together. So certain industries, banking, transportation, definitely manufacturing, mining, the end-to-end solution where the AI
40:04
works across all three and innovation is happening sort of synergistically makes more sense than a lot of the AI tools people talk about. Anyways, that’s number six. I think that’s enough for today. I didn’t really give you too many use cases, but they have some models out there, the Pangu railway model, where they’ve deployed Pangu to inspect railways.
40:31
You got all these railway lines across the whole country. You used to have to put teams of people basically riding the railways, checking for defects and checking for little things. Well, now you can put a basically a robot rail car with lots of cameras and it just zips along the railways all the time and can look for defects and do regular inspections. And, you know, it can catch pretty much everything and the cameras are getting better and better and the
41:00
The price is nothing compared to having people do it. They talk about things like they have some weather forecasting models, the Pangu weather model. You can look this up online. It’s pretty cool because they do have satellites in low Earth orbit looking at things. They have cameras on the streets, things like this. So, they can integrate the connectivity, the edge device, and the cloud, and the AI. And apparently,
41:29
I think this is true that some smart young guy at Huawei, like really young, like 22 or something crazy, figured out how to predict the weather in your neighborhood. Like, because you always see weather maps, but they’re for a city or a region, it can apparently tell you about the weather on your street. And it can predict if you’re going to get rain on your street or not. Which is really pretty awesome.
41:58
I mean, I haven’t played, I’m going to play with it because it’s available online. If I find the link, I’ll put it in the show notes. It kind of makes sense because the data already there. It’s just about making a better model that can do it. So yeah, there’s a weather model they talk about. They talk about smart mining. They talk about these railway models. They talk about manufacturing use cases. All of them are pretty cool. Anyways, that’s all fun. And there’s some others for like forestry.
42:28
and looking at Sichuan forests and the grasslands of China and sort of seeing how they’re doing things like that. There’s use cases all over the place. It’s pretty fun to look at what they’re doing. So, if I find the weather model, I’ll try and put the link and you can put your address and see if it works. Anyways, that’s what I wanted to cover for today. I thought it was just great talk and really well thought out strategy, but it also kind of my takeaway was
42:55
These two pillars, I’m calling them pillars, yeah, that’s really to me the frontier to pay attention to. This idea of sort of distributed cloud infrastructure and this idea of a cloud matrix and integrating connected GPUs, CPUs and all of that and sort of managing it in real time to increase performance. And the net result of all of this is to increase performance.
43:22
faster than rivals and to do that exponentially, which is better performance, but also cheaper, more efficient, more stable, lower latency. mean, there’s multiple dimensions here that kind of matter for AI cloud, especially for different types of companies like mining versus just document preparation would be very different. Anyways, that’s it for me for today. I hope that is helpful. I’m going to write all this up in some…
43:50
fairly dense articles and really sort of map it out. But yeah, and I’ll put the slides for those six points I just mentioned. I’ll put them in the show notes. You’ll have to click over to the webpage, ChefTowson.com. They don’t go into the Apple Podcast app, but they’ll be over there so you can click over and see it if you want. They’re pretty good. Anyways, yeah, that’s it for me. It’s been a crazy couple week. I’ve been bouncing around Phuket. I was up in Beijing just a real quick 48-hour trip.
44:19
to do some stuff there back again Phuket was pretty fun it’s a strange it’s a really interesting island it’s such a mix like I stayed in Karon which is one of the really amazing beaches on the west side absolutely beautiful beach I usually don’t go to Patong which is a little bit sketchy I usually go to Karon and Kata which are the two beaches just south of that beautiful beaches like we hang out we go swimming a lot it’s a lot of fun
44:49
The cities are not terribly nice. They’re basically kind of beach towns. And as far as I can tell, it’s like 80 % Russian now. Like, I’ve heard that there’s 200,000 Russians in Phuket right now. I don’t know if that’s true. It seems like it. If it is true, they’re all in Corona and Kata. Like, it is… You know, when I go to Northern Europe, Finland, Sweden, or Russia, I immediately become aware of the fact that I’m shorter.
45:19
Like I don’t feel shorter in Asia. I’m kind of medium height here. I definitely feel it in Sweden and Finland and Russia where everybody’s like 5’11”, the guys six-foot, six foot four. I’m walking down the street. I’m looking at the middle of people’s backs most of the time. women too, women are very, very tall and like Russians like 5’10”, 5’9″, 5’8″. I’m only 5’7″. And it was, that’s what Kata felt like to me in Corona. Like it was just tall white people.
45:48
And I’m staring at backs walking down the street. So that was a little bit strange but you know If I was a young man, I would probably have gone to Phuket too. I don’t want to get drafted. So totally sympathetic and then there’s weed shops about every four stores. There are weed shops everywhere. It’s a very strange place now on I Think a plus is if you want really good Russian food or really good Turkish restaurants
46:17
Katan, Karon have great Turkish restaurants in great rush. You can’t get those really anywhere else I know in Asia like this. So that was kind of interesting. Anyways, my side of the island, I go over to the east side Phuket town, Rawai, which is mostly Thai. It feels a lot more like Thailand and a lot less like the western side, which is kind of this international beach towns. But it’s great fun. We did the…
46:46
We basically just do water sports now, because the girlfriend is just every day, let’s go into the waves, let’s go swimming, let’s go, I don’t know, it’s just her thing. So, we went to the water park, which is called Andamanda, which is a really big water park in Kathu, sort of Phuket town area. Fantastic, it was the most fun. We’re doing water slides and…
47:10
the wave pools and the river, lazy river where you float. We spent hours just having the best time. So that’s kind of our thing is we go down and we just swim around and then we go to the water parks. Pretty spectacular couple days actually. So yeah, it’s an interesting place to go but pretty great. I think I like it more than Bali. Like there’s more to do and you don’t get the stomach. Your stomach doesn’t get bad because you ate contaminated food.
47:40
which is pretty much guaranteed if you go to Bali. mean, plan on spending a decent amount of the first 48 hours on the toilet if you’re going to Bali. So, there’s none of that. so anyways, that’s my own little bias about whatever. Anyways, that was it for me. Great time, then bounced into Beijing and bounced out of there pretty quick. So, it’s been a busy couple of weeks. Anyways, that’s it for me. Hope everyone is doing well. No recommendation, well, one TV recommendation.
48:08
Couple weeks ago. I rented I recommended a Netflix show called the Devil’s plan, which is the South Korean Sort of game show competition where you have to basically solve pretty advanced puzzles and pretty advanced games It’s like a high IQ version of Survivor or something like that. Anyways, they dropped the second season a week ago It starts off slow, but then it gets pretty good. So yeah, we’re watching that one. That’s pretty good
48:38
Anyways, that is it for me. I hope that’s helpful and I will talk to you next week. Bye bye.
———
I write, speak and consult about how to win (and not lose) in digital strategy and transformation.
I am the founder of TechMoat Consulting, a boutique consulting firm that helps retailers, brands, and technology companies exploit digital change to grow faster, innovate better and build digital moats. Get in touch here.
My book series Moats and Marathons is one-of-a-kind framework for building and measuring competitive advantages in digital businesses.
Note: This content (articles, podcasts, website info) is not investment advice. The information and opinions from me and any guests may be incorrect. The numbers and information may be wrong. The views expressed may no longer be relevant or accurate. Investing is risky. Do your own research.
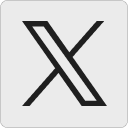



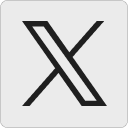
