
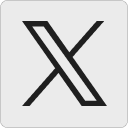

This week’s podcast is about my interview with Dongliang Guo, Head of International at Alibaba Cloud.
You can listen to this podcast here, which has the slides and graphics mentioned. Also available at iTunes and Google Podcasts.
Here is the link to the TechMoat Consulting.
Here is the link to our Tech Tours.
Here are my 3 take-aways:
- Take-Away 1: Alibaba Cloud is Focused on the Infrastructure and Model Layers. It Is Differentiating its GenAI with an Open-Source Ecosystem (i.e., ModelScope) and Model Building Tools.
- Take-Away 2: Southeast Asia Cloud Computing is Being “Fixed”. And Also Reshaped for GenAI.
- Take-Away 3: GenAI Has Surprising High Impact Use Cases Everywhere.
Cheers, Jeff
———
Related articles:
- GenAI Playbook (Step 3): How to Build Barriers to Entry with Intelligence Capabilities (9 of 10) (Tech Strategy)
- AutoGPT: The Rise of Digital Agents and Non-Human Platforms & Business Models (Tech Strategy – Podcast 163)
- Why ChatGPT and Generative AI Are a Mortal Threat to Disney, Netflix and Most Hollywood Studios (Tech Strategy – Podcast 150)
From the Concept Library, concepts for this article are:
- Generative AI
- Cloud Services
From the Company Library, companies for this article are:
- Alibaba Cloud
———transcription below
Episode 232 – Alibaba Cloud.1
Jeffrey Towson: [00:00:00] Welcome, welcome everybody. My name is Jeff Towson and this is the Tech Strategy Podcast from TechMoat Consulting. And the topic for today, three lessons from my interview with Alibaba Cloud. Now a couple weeks ago I was in Hangzhou and doing sort of tech company visits, which was super fun. And I did an interview with Dongliang Guo, who is the head of basically international business for Alibaba cloud, which was really kind of a phenomenal opportunity because this has been on my short list of, I mean, I’ve been following Alibaba cloud as closely as I can for a couple of reasons, which I’ll explain shortly, but.
You know, then to be able to sit down with the head of international and international is pretty much everything outside of China, which for Alibaba cloud is a lot of Asia, Southeast Asia, [00:01:00] Japan, North Korea, South Korea, not North Korea. And then, in addition to that, multinationals that are active in China, because, you know, these multinationals have to sort of.
Reconcile operations in two different geographies, standards, compliance, things like that. So, within this international business, Dongliang’s business, those two buckets of companies. So anyways, great opportunity. I’ll explain why I thought it was important. But yeah, I’m going to talk about sort of what my big takeaways were for that.
And that’ll be the topic for today. Standard disclaimer, nothing in this podcast or my writings or website is investment advice. The numbers and information from me and any guests may be incorrect. The views and opinions expressed may no longer be relevant or accurate. Overall, investing is risky. This is not legal tax.
Sorry, this is not investment, legal or tax advice. Do your own research. And with that, let’s get into the topic. Now, there are no real digital concepts [00:02:00] for today. This is about cloud services and it’s about generative AI and all the new tools and models. And, you know, a company like Alibaba Cloud sits right at the intersection of those.
I’ve been looking at really three of these companies in China, Asia, which are Baidu AI Services, Alibaba Cloud and Huawei AI Cloud, which is, it’s not as well known or as prominent, but it’s kind of coming up quickly along the outside, much more with a hardware focus as opposed to a software focus. Now, why do I like these?
Well, one, cloud services are generally good businesses, AWS, Azure, Google Cloud. They tend to be a very good on ramp, for trying new tools, trying new analysis, things like that. And they’re basically becoming the easiest way to get into generative AI tools. If you’re working on something like Google Cloud pretty easy to use [00:03:00] for those of you who aren’t software first people, which I am not as well.
You know, you get onto something like AWS or Google Cloud, you can very quickly learn how to sort of spin up a compute space and then start feeding in data and deploying apps. And, you know, if you’re really sophisticated, then you start customizing. So. For a lot of companies, you know, this is sort of the first step for getting into generative AI is let’s start building something on Google cloud Alibaba cloud.
If you’re in Asia, things like that. So generally, kind of a great space to look at. And I think people don’t pay as much or enough attention to the China giants, which are Alibaba cloud, Baidu cloud, Tencent cloud would be in there too, but I don’t really follow them as much. And then Huawei sort of coming up the outside.
Okay. Okay. Within that, Alibaba Cloud is sort of uniquely interesting for a couple reasons. One, it’s an e commerce [00:04:00] company first, and it’s a platform business first. So, you already have a huge number of merchants and brands. on the platform. So, it’s a very good platform for them to onboard. You know, if you’ve already got your shop on Taobao, Tmall, you know, to start adding generative AI tools is quite easy.
And that’s really their first vector in terms of getting adoption for their AI cloud services is their core e commerce business. So I’ve written about this before, but if you look at Alibaba’s generative AI strategy, not just Alibaba cloud, they’ll talk about things like chat bots for consumers and buyers on their e commerce platforms, which is true, but really I think most of the actions on providing tools for merchants and brands, content creation, customization, business insights, enabling you to go cross border with sort of business intelligence tools, which I talked about previously.[00:05:00]
So. That’s sort of the first stop for their generative AI strategy. From there, you get just general AI cloud services, which is another nice factor. And then the third thing I think they’re doing is deploying entirely new AI services that are really independent of their e commerce business. And the new CEO, Eddie Wu, I mean he’s basically talked about this when they laid out their big Alibaba strategy a year or so ago.
It was kind of going back to basics. Let’s go back to the basics of e commerce and platform building, because they build platforms. But in addition to that, let’s get into AI services. And the first one I think that they’ve really rolled out or is on the way is DingTalk AI, which is sort of their version of WeChat, which has always been more enterprise focused, so more like Slack or Microsoft Teams.
[00:06:00] And it looks like they’re going to turn that into a full-fledged AI service. With all sorts of, you know, agent AI and all these cool things. That looks like their first major one out of the gate, but we’ll see. I’m watching for this sort of new category of AI services that Alibaba wants to build.
Anyways, so that’s kind of the backstory. And based on that, when I was sort of in contact with them and they said, would you like to interview the head of our international business, I was, you know, absolutely, you know, anywhere, like, give me a call. I’ll be there. So. Let me go into a little bit about what I took away from this.
Now, my first takeaway was Alibaba cloud is focused and making, I think the majority of their big investments at sort of the infrastructure and the model layer. Now I’ve talked about this before, but when you map out the architecture for an AI tech stack, it looks, you know, it looks different than a, you know, a traditional sort of cloud tech stack, a [00:07:00] CPU based tech stack.
One, you know, you’ve got GPUs as pretty much the core of this thing. So, when you look at the lower levels, which, you know, generally referred to as infrastructure, you’re usually talking about cloud and you’re talking about semiconductors. So, NVIDIA has been doing spectacularly well because they, you know, they sort of dominate at the GPU level.
It looks like Alibaba cloud is focused on sort of the cloud compute layer. So, infrastructure and then one level above that, which would be the model layer. So, you know, they’re, they’re building, you know, a whole suite of large language models. These are sort of your L0 foundation models, which. started out with, you know, LLMs and then moved into image and video and multimodal.
And their latest version of that came out in September QN 2. 5, which is apparently getting good reviews. I’m not, I’m never [00:08:00] really sure how to rate the models versus others. There’s lots of metrics. There’s lots, people talk about it. I’m not sure I believe it. I’m, you know, I don’t have the expertise to really be able to tell which one is.
Inherently better. But you can usually look at uptake by developers. If you go over to Hugging Face or something, you’ll kind of see which models are getting the most usage by developers, which is a bit of a self-fulfilling prophecy often. So anyways, that comes out. Their Coder version, which is, you know, lets you write software.
Getting a lot of attention. So, sort of at the infrastructure level, cloud, cloud storage as well as the model layer. foundation models. That seems to be where they’re putting most of their effort and big dollars. Now that’s a little different than some. If you look at how Huawei talks about this, they will talk about their foundation models, their L0, but then they will talk about L1, L2, which [00:09:00] are customized models built on top of their foundation models.
So L1 is usually referred to as industry specific. The idea that you’re going to take a basic L0 model, let’s say like QN, and then you’re going to begin to, other people are going to customize that to make it specific to manufacturing. And then you could go up to L2 and you could make it specific to certain scenarios like customer service or finance.
which may not be as industry specific. So, you can see sort of customizations from L0 to L1 to L2. And then ultimately you get the app layer on top of that. It’s interesting to see where people are sort of placing their bets. Baidu is definitely playing the full stack and they had a bit of an advantage in this than that, you know, Robin Lee, 12, 13 years ago, running Baidu.
I mean, he’s an algorithm AI guy from the beginning. You know, he [00:10:00] was writing algorithms back in 1995, 1996 that, you know, Sergei Brin and them, they basically took his models and used part of that to create PageRank, which being became Google search. So, he’s been deep into AI his whole career. He made a big bet.
on the whole AI tech stack starting back 2012 ish. So, they’ve got their own semiconductors, they’ve got their own hardware, they’ve got their own foundation models, they’ve got the whole thing. That’s pretty unusual. Now that was a lot focused on autonomous vehicles for a long time, so if you go back five years and you looked at Baidu, what they were talking about was autonomous cars.
Well, then when generative AI dropped, they were incredibly well positioned. They pivoted over very quickly. They don’t talk about their cars that much anymore. Now they’re talking about all their generative AI tools and models and all of that. Anyways, Alibaba also incredibly well positioned, but you know, from what I can [00:11:00] tell as an outsider, more focused on the infrastructure and the model layer.
Less in terms of building out a huge suite of apps themselves. More like giving the tools to others and letting them then build out various apps. And I think that’s kind of their biggest differentiation right now. Is that, you know, they’re really focused on building an open-source ecosystem. Which is very different than say, like, OpenAI, where almost everything’s proprietary.
In contrast, Facebook is all open source, but You know, they’re not creating their own tools. They don’t look like they’re building a business there. They look like they’re more just creating tools, open sourcing them, and sort of scorching the earth in the profit centers of companies like OpenAI. Well, Alibaba cloud has clearly made a strategic decision that they’re going to create an open-source ecosystem.
They want everyone to build on their tools. Some of them are closed and more proprietary, but most of them appear [00:12:00] to be open source. And then they’ve got ModelScope and Model Studio, where you can build and share these models and all of that. So overall, that was kind of my first takeaway. They seem to be placing their big bets and their focus on the infrastructure and model layer.
And then not as directly doing all the apps themselves as some others, like say Microsoft. Okay. Let’s take away number one. Takeaway number two Southeast Asia. You know, that’s the big backyard of China. In addition, South Korea and Japan, but really Southeast Asia is where a lot of people are focused.
Dongliang, he talked about, I sort of asked, like, how are you, how are you getting adoption in Southeast Asia? What is your differentiating proposition versus let’s say AWS or Google Cloud? And when you look at the numbers who’s got market share really in Asia, but particularly Southeast Asia. In terms of infrastructure as a service, Alibaba Cloud is number one.
Right, everyone [00:13:00] Alibaba Cloud when you look across Asia. That’s arguably number one or number two depending on what metrics you look at. So, I kind of asked, how do you differentiate? Takeaway number two was it seems to be a focus on fixing current cloud deployment and then reshaping the tech stack for companies.
So, the example he gave was, you know, the cloud is not new to Southeast Asia, but a lot of companies deployed. They started shifting some of their workload from on premise up to the cloud. And you know, the, the, the promise of the cloud was it’s going to be scalable. It’s, you know, you can use as much as you want when you need it.
You’re not going to max out your servers. It’s flexible. You can scale up and down depending on how much workload you’re dealing with right now. And it’s efficient, right? The cost is a bit less. There’s no major upfront cost like [00:14:00] You know, building a server farm in your business. That’s always been the promise of sort of cloud services.
Yeah, apparently there’s a significant number of businesses in Southeast Asia that never really got those benefits. Maybe they deployed on the cloud, but they never really scaled down their on-premises staff and capabilities. Maybe they’re not moving their business forward. There’s sort of usage up and down with volume.
They’re just sort of paying more than they should be. So, there’s this idea of fixing current deployment. That’s my words, not his. Actually, none of this I’m saying is, is his words. This is all my interpretation and my summary. So, it’s the idea of going into people who’ve already deployed and fixing their deployment.
That would be the fixing part. And then the other part is, okay, now we’ve got to reshape your tech architecture. Because when you start wanting to deploy generative AI tools, well, you’re going to need a model. Well, traditional cloud services and traditional on-premise tech [00:15:00] architecture don’t have models.
And also, they don’t usually have a sufficient data layer where you’re going to see large volumes of data flowing all the time to keep your models functioning. So, you start to basically reshape the tech architecture at a lot of companies. You know, unfortunately, well, maybe that’s not the right word.
Unfortunately, generative AI does require a parallel tech stack. You can’t just use your CPU based stack to do this stuff. You’re going to have to build out the GPUs, the model layer, the data layer, the customizations, the apps. So yeah, that’s just kind of where we are. But, anyways, reshaping is a pretty interesting opportunity in Southeast Asia.
A lot of companies are starting to do that. I would say most of them are still at pilot level. You know, you [00:16:00] sign up for something like AI Cloud with Alibaba. You start to build out a customized model on the compute that you’re taking from them. That is a involves feeding in a lot of your data, which you may not have enough of it.
You may have the data internally. Most companies don’t. You may have to sort of partner with others and do data sharing or a data ecosystem with other companies. Maybe your compliments, maybe other industry players, but you’ve got to get the data flow up. Most people don’t have enough internal data to do this.
And then you start to sort of flood that into your models. That gets you a bunch of pilots. I encounter a lot of companies that are sort of stuck at this level. They’re sort of what we call pilot purgatory. And you really want to push past that and start to scale up one or two pilots and get them deployed at scale.
Because that’s when you’re going to start seeing business results for all this effort. So, yeah, getting out of that pilot purgatory is a bit of a challenge often. [00:17:00] Anyway, so that’s kind of what they talked about. Takeaway 2, Southeast Asia. A lot of fixing current cloud deployment as well as starting to reshape to a generative AI world and then you try and scale up as much as you can.
One point I didn’t mention, one of the things he mentioned that was interesting when we were talking about infrastructure was, okay, how is your cloud service infrastructure different for generative AI than others? And one of the things he mentioned that I thought was really interesting was the need for stability.
and flexible allocation of resources. Now, you know, when you start doing your models, you know, phase one is you’re training the model. Okay, it turns out stability is kind of a big deal. because you’re investing a lot of time and effort, you know, weeks, months to train your model and get it to a certain level of accuracy.
Now if you’re doing that on a cloud [00:18:00] service and your cloud is unstable or going down five, six, ten times a day, you can significantly increase the amount of time it takes to train. So, he had mentioned that which I thought was interesting, that in the training phase, the stability of the provider is actually kind of a big deal because it directly relates to how long this takes and therefore how expensive it is.
I thought that was kind of interesting. The other point he made was it helps if the resource allocation, your cloud resources, are flexible because eventually you move from training to inference. And training is much more predictable. You kind of know how long it’s going to take to train your model, how much compute it’s going to require.
Ongoing inference, running of the model, is much less predictable. And it helps if you are able to sort of shift your compute resources, which you’re paying for, between [00:19:00] training and inference as you need. And he mentioned that’s something that Alibaba Cloud offers, that you can sort of tie these two together and then shift your resources as you need.
Very interesting. I didn’t know that. Okay. Last lesson, basically that e commerce and digital natives are the major, you know, sort of early adopters of all of this stuff. Actually, it was interesting. I kind of said, well, who’s, who’s really adopting? Cause all this stuff is interesting in theory. There’s a lot of piloting going on, but you know, the key question is like, look, who’s deploying at scale and seeing real results?
That’s what we want to see. And you know, the standard answer to that question is the digital giants, the digital natives, the hyperscalers, which e commerce companies are a big part of them because they’re sort of born digital. So, they’re already set up that way. Deployment is a lot easier than let’s say you’re a supermarket trying to adopt this stuff.
So that wasn’t a surprise, but then he kind of said something interesting. He said, actually they weren’t first. [00:20:00] The gaming companies were first. Smaller, but actually several months ahead. And I thought that was kind of interesting. And the example he gave was You know, when you’re, you’re developing a video game, you know, the first step or one of the early steps is master design.
You do a lot of artistic work, you design your characters, what they’re going to look like, the key players, the key story. But there’s a visual aspect to let’s look what all the players look like. But then once you get the master design, then you have to build lots of versions of these same characters, different outfits, different scenes different weapons, all of that, whatever you’re playing.
And he had mentioned that actually generative AI is quite good at that second step, that once you feed in the master design, it can iterate and create all the secondary versions very easily. Well, not easily, but more efficiently cheaper, faster. So. Yeah, that was kind of interesting. [00:21:00] I had spoken with NetEase a couple weeks ago and had asked them the same question, what are you using generative AI for?
And usually when I ask this question, the first answer you get is we use it for internal productivity. You know, we take our highly trained staff that are usually quite expensive and we make them a lot more efficient, effective, productive, basically. But then beyond that That’s usually step number one for Gen AI strategy.
Step number two is then we start to change the product itself. So maybe we start to put battle robots in play or we start to put NPCs that are intelligent. Okay. Now you’re changing the customer experience. That’s a little bit riskier because if you screw it up, it wrecks your product. So, most people start with productivity tools.
I know. So, gaming turns out they were first after that e commerce, other digital giants, The hyperscalers. I’ve mentioned a couple of those that, [00:22:00] you know, within e commerce you’re going to be hesitant to deploy this stuff as a chatbot. You might do a little bit, you might do it for customer service or something like that, but you’re not going to deploy these things as your primary user interface because it’s probably not good enough yet.
There’s risks, but you can definitely give your tools to merchants and brands and let them start doing things like content creation. So, if a merchant, a sneaker merchant on Taobao is taking photographs of their shoes, you can upload them and it will create all the content that goes in the product description under them.
Right? Things like that. If you’re creating lots of marketing, well Generative AI is good at creating 10 more versions of what you already did and then you can choose the best one. So, a lot of content creation like that we’re seeing merchants and brands do that. Now I think the interesting question here is high [00:23:00] impact versus low impact versus medium impact.
And the interesting bucket within all of this is what are the high impact use cases? And he mentioned a couple that were interesting. Within e commerce the one that he mentioned was B2B e commerce. for sort of almost industrial products where there’s a huge number of them. And the example he gave was screws.
If you are selling screws to construction companies, do it yourselfers, all of that, one, they don’t cost much, so very low-ticket item, but there’s a million types of them. It’s never really been worth it. To have people take photographs of every single screw and then fill in all the product information online, the size, the make, where it’s from, shipping.
I mean, it’s too much work for these little items [00:24:00] of which there’s a gazillion of them. So, he had mentioned, you can basically use generative AI, show them to the camera and the AI will fill in all the product information you could ever need for every single individual type of screw. That’s kind of interesting.
Now is that high impact? No, I would put that as medium impact. But it’s going to play out in a couple of ways. When you fill out more of these data fields, suddenly the search on your site is going to get better because the search is dependent on the data fields. Okay, that’s kind of interesting. The recommendations are obviously going to get better.
And then, you know, third, you’re going to get better business intelligence reports, things like that, to management. And that was another use case he brought up that I thought was really cool and is something I’ve been thinking about a lot, which is, how do you use generative AI to make your management team smarter?
And your typical management [00:25:00] team has been doing their business for 20 years. A lot of expertise in their minds. They’re able to make decisions very, very quickly. And the way things have been working for the last 20 years is then you give those senior people. more and more data. You give them a dashboard, you give them metrics, and you know, you combine their expertise with these sort of data systems.
However, those data systems are largely based on structured data, numbers, but they’re not based on photos, they’re not based on conversations. All that sort of unstructured data, you know, it’s, it’s a big mess. You know, management tools, their standard dashboards are based on data warehouses, not data lakes.
Well, it turns out generative AI is very good at looking at things like all the conversation this customer has had with the company, whether it’s customer service or chatbots or whatever. That’s lots and [00:26:00] lots of conversation. Very unstructured data, messy. Well, generative AI is very good at doing that.
Zipping through that and gleaning out sort of takeaways and various intelligence that you can then feed to the management team. So suddenly you’ve got three sources of sort of expertise and decision making. You’ve got the internal expertise of the managers themselves. You’ve got structured data often on dashboards, and then you’ve got this new source of intelligence coming from all the unstructured information.
All the, all the data. All the videos, all the conversations, all of that can get distilled into real insights. Yeah, that can make your team a lot better. I thought that one was really interesting and I think that’s sort of a high impact use case. So anyways yeah, that’s basically takeaway number three is, yeah, the digital giants went first, e commerce especially, but there’s a lot of these high impact use cases [00:27:00] popping up all over the place and often in places you wouldn’t expect.
Management dashboards, industrial e commerce, the back office of healthcare companies, the back office of banks. Like one thing we talked about was like, if you’re in a pharma company, you have incredibly highly trained and very expensive people doing a lot of report writing, you know, because there’s a lot of compliance requirements.
Well, suddenly you’re spending very expensive people are spending a lot of time just basically doing paperwork. You give them a generative AI tool; you get a big bang for your buck on stuff like that. So, there’s these high impact use cases kind of all over the place. It’s really interesting and I’m trying to dig into more of those.
Just create master lists of all of these random use cases that get you sort of a big ROI on your time spent and money. Anyways, those are kind of my three [00:28:00] takeaways for the visit. Really cool. I guess one last disclaimer. All of this is my interpretation and my takeaway, so none of this is a quote from Dongliang or anyone at Alibaba.
So yeah, this is all just me and what I summarized, which is not necessarily the same thing. In fact, For sure it’s not. Anyways, that is the content for today. I hope that’s helpful. As for me, I’m in Northern California for the next week or so. It’s seeing the family for the holidays and all that. And the weather is absolutely miserable.
It’s really unpleasant. It’s cold. It’s rainy. You’ve probably heard the rain during this podcast. It’s been on the roof. Yeah, I’m not enjoying this at all. Well, I’m obviously enjoying seeing the family, but I think I’ve lived in Asia and Southeast Asia too long, like I have whatever little tolerance I had for the cold is completely gone.
I don’t like [00:29:00] this. I’m just working from home. I’m not going outside. Getting a tremendous amount done. So, I suppose that’s good. But yeah, generally it’s that’s been my week. So actually, it’s kind of interesting. Northern California, it’s, you know, it’s just land of the Teslas. They are absolutely everywhere.
I’ve seen like Three or four Cybertrucks in the last day cruising around. This is in the Bay Area, Northern California. I saw one bright blue one, which I didn’t know they made them in colors. Every time I’ve seen the Cybertruck, it’s that sort of, you know, metallic steel, silver. I saw a bright blue one, like shiny blue, like royal blue.
It was kind of ridiculous. I saw a matte black one, which was pretty cool. Pretty cool looking. That one was pretty cool. I can kind of get that. Yeah, they’re, they’re interesting. I’m going to go down to the Tesla dealership after this, when I’m done with this and see if I can sit in one and explore the inside.
I, the, what, the one thing I did notice is they’re much bigger than I thought they were. [00:30:00] Like there’s something weird where if you look at a cyber truck, it looks big, but it’s, it somehow looks smaller than it really is. When you stand next to them, I mean it goes above my head pretty much. The wheels are absolutely huge.
To get into the thing, you basically have to climb in it like a bunk bed. There’s something weird that they don’t look as big in the photos as they are in real life. I’m not sure. Maybe it’s the odd shape or something like that. I don’t know. Anyways, that’s what I’m going to do this afternoon. I’m going to go down and see if I can get a They don’t let you drive them, but you can sort of play around inside.
So anyways, that’s my plan. Okay, anyways, I hope this is helpful and I will talk to you in a couple days. Bye. Bye
——-
I write, speak and consult about how to win (and not lose) in digital strategy and transformation.
I am the founder of TechMoat Consulting, a boutique consulting firm that helps retailers, brands, and technology companies exploit digital change to grow faster, innovate better and build digital moats. Get in touch here.
My book series Moats and Marathons is one-of-a-kind framework for building and measuring competitive advantages in digital businesses.
Note: This content (articles, podcasts, website info) is not investment advice. The information and opinions from me and any guests may be incorrect. The numbers and information may be wrong. The views expressed may no longer be relevant or accurate. Investing is risky. Do your own research.
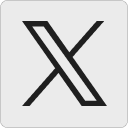



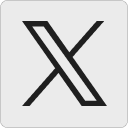
