
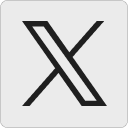

I have argued there are 4 pillars to Baidu’s AI Cloud strategy – which are:
- Pillar 1: Baidu AI Cloud Is an Innovation Platform Pushing to Reach Critical Mass
- Pillar 2: Baidu AI Cloud Is Also Moving to Become a Technology Standard Deeper in the AI Tech Stack
- Pillar 3: Baidu’s AI-Focused Cloud Has 2 Advantages Over Other Innovation Platforms
- Pillar 4: Baidu’s AI Cloud Is a Flywheel in Industry-Specific Intelligence
I covered the first three pillars in Article 3.
Which brings me to the final pillar of their strategy. And this is my favorite question in all of this.
This is how Baidu describes the advantages of its AI cloud business:
“The core advantages of ERNIE lie in its knowledge enhancement and industrial-level application.”
That’s the key sentence. “Knowledge enhancement” plus “industrial-level application” is the important concept.
Here’s more from Baidu. I added the bold.
“The (ERNIE) model learns from large-scale knowledge maps and massive unstructured data, resulting in more efficient learning with strong interpretability. It also aims to promote the intelligent upgrading of industries by constructing a foundation model system that is more suitable for specific scenario requirements. This includes providing tools and methods to support the entire process and creating an open ecosystem to stimulate innovation.”
Recall the graphic I showed earlier.
You can see the four levels of their AI tech stack.
- Chips (i.e., Kunlun) and compute (i.e., servers). Based in the cloud.
- Deep learning platforms for developers (i.e., PaddlePaddle)
- A suite of big foundation models (i.e., Wenxin / ERNIE)
- Industry focused applications.
I would actually add another 2-3 layers to this tech stack.
- Databases and data ecosystems. Above the chips, servers, and compute, you need massive amounts of data being processed and shared.
- L1 and L2 models. Huawei has a nice breakdown between big foundation models (L0) and customized industry specific models (L1) built from them. And then applications (L2) above this.
- A token processing layer? Chamath Palihapitiya has been talking about Groq as a new part of the AI tech stack. Basically, a layer that provides low latency, low-cost token processing. So, AI can be more interactive.
Now look at the arrows on the left and right of the graphic. That is the flywheel Baidu is talking about. The flywheel goes between model intelligence, performance and efficiency and industry application and usage.
- The more users, use cases, apps, APIs, and data coming from industry usage, the more accurate and efficient the models will become.
- And more intelligent and efficient models will encourage more usage, apps, and use cases.
It’s a flywheel in industry-specific knowledge.
And CEO Robin Li has been quite vocal about the need to make generative AI useful in practice. And specifically, useful in industry applications. Li has stated that creating so many LLMs (which China is doing) is a waste of time. The key is to focus on applications and use cases. And build backwards from there.
Read that key phrase about ERNIE again.
“The core advantages of ERNIE lie in its knowledge enhancement and industrial-level application.”
We know what industrial-level application is. But what is “knowledge enhancement”?
The Engine of Baidu’s AI Cloud Is Industry-Specific “Knowledge Enhancement”
How do humans learn?
We consume lots of data and information all day long. We see the real world. We read. We listen to podcasts. And so on.
But we also depend on an internal knowledge graph that is combined with constantly inflowing information. We need a way to efficiently and effectively process and understand all the data coming in. So, we have knowledge graphs that are passed down and inherited. I think of these as internal frameworks. Some of our knowledge is innate. Language seems to be hardcoded into humans. But most of our knowledge frameworks are passed by schools and training.
That simplistic analogy is how I think about knowledge graphs in machine learning. But I could be wrong about this. I am still trying to understand the mechanics of how this works.
My working (and simplistic) understanding is that the efficiency and effectiveness of machine learning is ultimately going to depend on big foundation models that have both tons of data and large knowledge graphs. That’s “knowledge enhancement.” I think.
Note the above quote again.
“The (ERNIE) model learns from large-scale knowledge maps and massive unstructured data, resulting in more efficient learning with strong interpretability.”
Baidu’s plan appears to be to combine industry-specific knowledge graphs with massive amounts of unstructured data, coming from on-the-ground industrial use.
Baidu has recently said it now has the world’s largest knowledge graph at a scale of 550 billion. I’m not really sure what they mean by this. But, according to Baidu, their big model ERNIE is advancing with much better efficiency and output than models that don’t have “knowledge enhancement”.
Robin Li actually gives presentations that are very specific about how they are measuring the intelligence of their solutions. He breaks intelligence down into understanding, generative skills, reasoning skills, and memory. At the October Baidu event, he gave live examples of ERNIE in each of these areas.
- He tested ERNIE’s understanding by asking about public housing loan policies.
- He tested its generative skills by producing advertising posters, copy and videos.
- He tested its reasoning skills with math problem.
- He tested its memory skills by having it write a martial arts novel. Not only did it draft the outline. It could repeatedly draft new characters and conflicts.
These are the four areas where I’m watching the performance improve versus other solutions.
Where the Rubber Hits the Road: 4-5 Flywheels in Industry-Specific Intelligence
Ok. That was some of the mechanics. Which I am really still learning about.
But where does this matter in practice? Where does the rubber hit the road for Baidu’s AI Cloud solutions?
Basically, 4-5 flywheels in industry specific intelligence.
The strategy is to increase use by industry, which creates better and better models and knowledge graphs for those specific industries. Knowledge enhancement is industry specific. Here’s how Baidu presents it.
So, we can look at the various industries and track adoption and performance over time.
The one I am most interested in is Kaiwu, their “Smart Manufacturing Platform”.
Kaiwu was launched in 2021 as an industrial internet platform powered by AI cloud. Given China’s massive operational footprint in manufacturing, it’s not surprising this is a focus. It’s a really big opportunity. And we should 100% expect a China-based cloud company to win in this sector.
The Kaiwu pitch has been about improving quality and efficiency of factories. And accelerating digital transformation. Ok. Fine. That’s a standard cloud services value proposition. But this has now been upgraded to focus on “intelligent transformation”. And to lower the threshold for AI applications in the industrial space. This flywheel depends on adoption. So, lowering the threshold for adoption is a strategic priority. And Baidu says they are now providing services for +180,000 industrial enterprises.
Here’s a one-pager for Kaiwu.
The other industry flywheels they talk about are smart finance, smart energy and water management, smart cities, and healthcare.
Ok. Let’s finish up this article series with competitive advantage.
Last Question: Is “Knowledge Enhancement” a Competitive Advantage? Is it a Network Effect?
As mentioned, Baidu’s AI Cloud is an innovation platform at its core. And this can mean big network effects. So that is the competitive advantage that matters most. Whether this develops or not depends on users, developers, APIs, and apps deployed. Those are the key metrics to follow right now.
But does the discussed knowledge enhancement flywheel create another network effect? Another moat?
If you have larger data flows and a more developed knowledge graph than your competitor, you should greater knowledge enhancement in your service. Does this get you greater efficiency and effectiveness for users? Does it mean a faster rate of advancement? Those are the metrics I would be looking for.
However, in the short-term that is not a network effect. That is superior operational performance. That’s usually what you get with a flywheel. You get superior operating performance for a period of time.
Here’s how Baidu describes the impact of knowledge enhancement and the generalizability of big models.
“Big models demonstrate effective generalization potential, allowing developers to create AI models at a much lower cost and threshold, accelerate a wider application of AI across industries.”
That’s important. Big models are generalizable, and this lets you create customized models faster and at a lower cost.
That’s also not a network effect. That’s economies of scale on the production side.
Baidu also asserts that big models have “been able to improve their knowledge scale while benefiting from reduced learning costs.”
Ok. That’s interesting.
I’ve been thinking about rate of learning as an operational advantage and maybe a competitive advantage for a long time. Here’s what Baidu says.
“Self-supervised learning from these data provides the big model with general knowledge alongside better adaptability and application efficiency. In practice, fine-tuning on top of pre-trained big models is creating a more unified and streamlined AI training system.”
“This approach has allowed big models to be flexibly customized on a large scale with zero or small samples to produce better results tailored to a variety of tasks.”
They are talking about a system that can learn faster. Can adapt better. And with less cost and less data. That is literally the definition of rate of learning and adaptation. Which I put as the L in my list of digital marathons.
So, we can see:
- A flywheel in operational performance.
- Some degree of economies of scale in model production and customization.
- Maybe an advantage in rate of learning as a digital marathon.
But is rate of learning showing up as a competitive advantage?
I’m not sure. This is literally my #1 question in digital strategy. Is Machine Learning turning rate of learning into a competitive advantage?
Here’s what I see today for competitive advantages for Baidu AI Cloud:
- Network effect on innovation platform
- Economies of scale in:
- IT fixed operating and capital costs
- Data
- Model production cost
- Maybe data as a scare resource
- Maybe IP and Proprietary technology
It looks to me mostly like a network effect and scale advantages in industry-focused intelligence models. This scale advantage comes from superior scale in inbound data (a data scale advantage). But also, from a larger knowledge graph, which we can characterize as a scale advantage in data or learning. Or as a scarce resource or proprietary technology.
But like all economies of scale, it does not necessarily go on forever. You want to see if this is durable. In a simple industry like soda manufacturing, I would not expect a scale-based knowledge advantage to persist over time. It will flatline and become table stakes over time. But you could see this advantage persist in complicated or rapidly advancing industries (like automobiles).
That’s my current take.
Hey, What About Apollo Go and Autonomous Driving?
There is one topic I have not really talked about. Baidu has been making big moves in intelligent driving for a long time. It is one of their three business units.
I view this as another industry vertical in Baidu’s AI Cloud strategy. Intelligent transportation and infrastructure are a vertical.
And intelligent transportation quickly becomes about intelligent driving. And autonomous driving. And mapping.
***
Ok. That’s it for Baidu, which I know has been kind of a lot. Congratulations if you got through all four articles (and 2 podcasts).
Cheers, Jeff
——-
Related podcasts and articles are:
- Baidu Is Externalizing and Exploiting AI. But It’s All About the Cloud. (Pt 3 of 3) (Asia Tech Strategy – Daily Update)
- Can Baidu Thrive As a Stand-Alone Search Engine? (Asia Tech Strategy – Podcast 76)
From the Concept Library, concepts for this article are:
- AI
- Generative AI
- Cloud Services
- Rate of Learning
From the Company Library, companies for this article are:
- Baidu: AI Cloud (ERNIE, PaddlePaddle, ERNIE bot)
——–
I write, speak and consult about how to win (and not lose) in digital strategy and transformation.
I am the founder of TechMoat Consulting, a boutique consulting firm that helps retailers, brands, and technology companies exploit digital change to grow faster, innovate better and build digital moats. Get in touch here.
My book series Moats and Marathons is one-of-a-kind framework for building and measuring competitive advantages in digital businesses.
Note: This content (articles, podcasts, website info) is not investment advice. The information and opinions from me and any guests may be incorrect. The numbers and information may be wrong. The views expressed may no longer be relevant or accurate. Investing is risky. Do your own research.
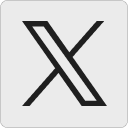



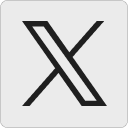
